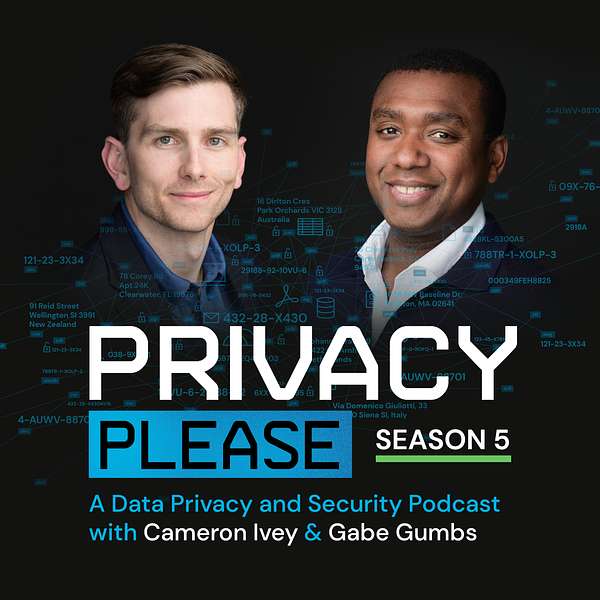
Privacy Please
Tune into "Privacy Please," where hosts Cam and Gabe engage with privacy and security professionals around the planet. They bring expert insights to the table and break down complicated tech stuff everyone can understand.
Privacy Please
S5, E222 - Navigating Privacy Challenges and Future Tech with Aaron Weller from HP
Unlock the secrets of privacy innovation and assurance with our enlightening conversation featuring Aaron Weller from HP. Aaron walks us through his fascinating journey of establishing a new privacy engineering function at HP and expanding into privacy assurance. You'll discover how his team is tackling significant challenges, such as developing standards for data aggregation and preventing re-identification attacks, with practical examples like employee surveys and website performance metrics.
What happens when the need for privacy intersects with the rapid advancements in AI? We delve into this critical topic by examining the dual threats of data re-identification and evolving AI legislation. Aaron provides invaluable insights into the ethical principles and security measures necessary to navigate this complex landscape. Additionally, we give you a sneak peek into an intriguing upcoming panel on AI governance featuring a humanoid AI, highlighting the unpredictable and exciting future potential of this groundbreaking technology.
Looking to the horizon, we explore the future predictions in data governance and the revolutionary impact of quantum computing on cryptography. Aaron discusses the strategic rethinking required as AI and technology advance, emphasizing the importance of integrating privacy measures directly into the code. Join us as we reimagine our relationship with data, ponder the necessity for solid foundational frameworks, and highlight the critical role of early detection in privacy issues. This episode is a must-listen for anyone invested in the future of data management and privacy.
Gabe, it's all right. Oh, does everyone know? Does everyone? Because I moisturize, and need I remind you it does not crack. We are live, though, so that happened, we are.
Speaker 2:Well, ladies and gentlemen, welcome back to Privacy, please. Cameron Ivey, here hanging out with Gabe Gumbs and a special guest today, aaron Weller. He's the leader in privacy, innovation and assurance COE at HP. Aaron, thanks so much for being with us today.
Speaker 3:Oh, no problem, Thanks for inviting me back.
Speaker 2:Yeah, second time we had you back a couple of years ago now, when you were kind of running your own company and doing stuff there there's been some changes since own company and do. There there's been some changes since um. Would love to hear about what, what you've been up to and maybe for the listeners as well, what's going on in your life right now sure?
Speaker 3:so, yeah, I'm coming up on two years at hp, so it must have been a couple of years ago, uh, we last chatted, but uh, yeah, so I joined back in the end of 22 to uh to head up kind of a new privacy engineering function, uh, which is something that I stood up. I was the first person in that team and stood that up, hired a few people and then, fairly recently, my role has expanded a bit. So we're now going to be standing up a privacy assurance team as well that I'm going to lead, as well as expanding kind of rebranding more than expanding kind of the engineering function to cover a lot of the innovation that's going on at HP. Right, we've got a long history of innovation and I thought engineering doesn't really kind of cover all of the stuff that we're doing, particularly when you get into the AI space. So still the old stuff, but a bunch of new stuff as well. It's incredible.
Speaker 1:What are you most jazzed about? Because this is definitely a significant undertaking that sounds like it is filled with a lot of fun, but also a lot of challenges. What are you jazzed most about?
Speaker 3:Yeah, I mean I think I tell people I get to work on the fun stuff. My team is looking kind of into the future and how do we cope, you know, cope with stuff and digest things before they become problems. So a lot of the I would say the wins that we've had are when we can actually find a solution to a problem. That wasn't necessarily obvious and it needed a bit of digging to get there. So even some of the work, I mean it sounds simple but one of my team really recently published a paper around aggregation techniques where there's remarkably little in the literature about aggregation for privacy.
Speaker 3:There's a lot around kind of statistical analysis and that kind of thing, but really applying that privacy lens to something that you think is it's a basic technique but it's so commonly used that you know having a standard for how do we do that commonly used that you know having a standard for how do we do that. You know how do we judge the risks versus kind of what we're actually aggregating. You know all the way up to then. You know some of the stuff we're doing around AI use cases and really you know how do we look at what those risks are as they're emerging and kind of as the standards are being developed to my team in some ways is helping to develop those standards Right. So I think a lot of it comes back to just the chance to go and solve problems, which is something I've really enjoyed throughout my career.
Speaker 1:I am intrigued by the aggregation problem. We don't have to dig into it now, but I feel unfamiliar enough with it that I'm almost compelled to clarify it for the listeners as well.
Speaker 3:Yeah, so it comes up in a number of different areas. So you could be aggregating, like a common use case would be with surveys, right, we do a survey. What is the? If we want the respondents to remain anonymous, what's that minimum aggregation limit? But if you're looking at kind of tabular data some different kind of there's more richness to that data. So potentially you've got more avenues of attack. So we're really looking at some of these things for a privacy attack perspective.
Speaker 3:How would you identify an individual? How would you single out that person within that data set? You can imagine that with employee surveys. Right, we know something about the employees as well. So if you don't have a minimum size, it's fairly easy to say, oh, that must be Joe, because you know, I know how Joe writes and that's how kind of they answer the question.
Speaker 3:So it's everything from kind of that kind of survey-based stuff to then when we're looking at kind of getting more, you know what's the performance of this website or this app and we're getting more of those aggregated statistics. So, yeah, lots of. When you get into the actual data itself, that's where you start getting some of these different nuances, and that was really where the research was focused is what's out there in the literature. A lot of the stuff that's out there publicly is around health data right, because a lot of that data is then published as research studies, but you don't want to identify the people who are part of that. So try to take that health data because of course, we're not a health care company and how do you then apply and generalize some of that stuff to the kinds of data that we work with?
Speaker 1:So now you're talking my language. You see, this is the problem as someone who just thinks purely as an attacker More days than not You're. You're thinking about it very much from a defender's standpoint, which I get now that you say it that way. So what you guys are looking into are novel ways to prevent re-identification attacks, exactly.
Speaker 3:Yeah, so re-identification is a big part of. I actually have someone who's pretty dedicated to re-identification attacks. And then how do you calculate some of the probability of that re-identification being successful? So we're doing a study at the moment, for example, around different synthetic data generators and can you compare their re-identifiability depending on kind of how they generate that synthetic data from real data? So, yeah, a lot of it's really getting back to how do we go away from this very fuzzy concept of risk that a lot of people use and really being able to quantify some of those things using kind of engineering and stats, to imagine that the person that does this full time on your staff.
Speaker 1:There's all of these other aspects of re-identifying data by itself, aspects of re-identifying data by itself. It's obviously, by definition, not really possible, because if it's been de-identified and that's all you have, sure. But then you start adding different source data to it and that's where you start getting the crossover in those tuples, but that seems almost like it might be. Well, it's a hard problem. Let's start there, which is why I presume your team's on it, because it's sure it's fun. Well, it's a hard problem. Let's start there, which is why I presume your team's on it, because sure, it's fun, but this is a hard problem. You don't know what you don't know, and you also don't know what data sets might be released, by whom and under what circumstances, including those in the dark web, for example, that could be used to re-identify data.
Speaker 3:Yeah, all of those things go into the threat model that we've developed. So we're looking at, you know, is it just going to be used internally? We're going to be publishing this stuff externally. Is there the possibility of a data set that you could, as you say, kind of recombine? Because if you remember back in the day, right, the Netflix example, aol search results, right, there were these data sets where you had the same people in the data set.
Speaker 3:The good news for us is a lot of our data sets are really about the devices that we sell, so there isn't really a direct compare. It's not really that traditional, you know what you'd think of as personal data for the most part. So that does mean it's a harder problem for someone to attack because they don't really have a. There's no good starting point for them to perform an attack against the data set, unless they've got something else. But, yeah, all of that stuff goes into our threat model and then we can kind of make determinations based on those different factors. And, as you say, you don't know all of this stuff, but you can make a pretty, you know a pretty solid set of guesses that give you at least some outlines of where you want to be.
Speaker 1:This is fascinating. I do not know how much of this work will make its way well, the substrate of the work but I'd love to get a better understanding of what a re-identification threat model looks like through the eyes of someone who's researching it daily. That would be of high interest to me, just from a personal kind of.
Speaker 3:Yeah, it's. One of the real interesting things for me is that when my team go to conferences and they're like other people aren't thinking of this stuff or we're further ahead in kind of the thinking than some of these other folks, which is it's always gratifying that we're along the same track and oftentimes it's having that person who can go and dedicate time to, to really thinking about these hard problems right, as opposed to me being scattered in 50 different directions. It's nice to be able to give people a smaller set of problems and say go and work these until you get to a good point.
Speaker 1:That's awesome. That's really cool. I am super fascinated by this particular. I mean for what it's worth, full disclosure, excuse me, I mean for what it's worth full disclosure, excuse me. Right before COVID, we'd set out to do some research in this area ourselves, just some very light kind of side work, and I just never got the cycles to finish up the work.
Speaker 1:But the theoretical portion of it, having done a bit of looking on, excuse me, I feel like the threat of re-identification is growing and growing. I mean, I don't know how you guys feel about it, but I don't think that there's two things. I think that there's two dynamics. The first is, to your point, a lot of people just don't seem to be thinking about it. And then the second point is there are a lot of people thinking about it and they're data brokers. They are. They're all about re-identification attacking Excuse, people thinking about it. And they're data brokers. They are. They're all about re-identification attacking, although, excuse me one, I don't know if you would call them re-identification attackers, although that's a. That's a better name than data broker. Data broker makes it sound like they're in the. It's some kind of legitimate business what do you mean?
Speaker 2:that's not a legitimate business I mean so is so?
Speaker 1:is, you know, la costa de nostra? Hey, you didn't hear it from me.
Speaker 2:I'm still sitting on the part, aaron, where you said that HP wasn't a health company. I'm just my goofy self just thinking that H and HP being health obviously is not to the Pack backward, but still, what do you think the biggest challenge in AI today is just, generally speaking, maybe from all your research that you found interesting, or maybe something that you didn't realize.
Speaker 3:Yeah, for me I think that the challenge is and even just it's keeping it. I've read a couple of articles this morning and I just got off a call about some of the AI legislation landscape. There's so much going on that just trying to understand you know, what are the things that that I should be concerned about, and I was giving a presentation yesterday that someone asked me the question how do you keep up? A lot of it comes back to the principles we just published externally our ethical principles, which you can find on our website, and really I think having that strong foundation of what we're about when it comes to AI, it helps to make sure that when we're looking through the lens of these new laws and things that are coming out, we've got kind of those foundational things we can always come back to around transparency, fairness and those kind of things.
Speaker 2:Yeah, that's probably the trickiest part is with all these new laws coming out. I think there's a slew of them in the next few months. I know the Montana one's coming out October 1st. When it comes to privacy and AI, when you're researching and going through things with your team, how do you balance that innovation without sacrificing that risk?
Speaker 3:Yeah, it's trying to go through and say what are the questions that will tease out some of those risks? Like, without going into the nth degree, about vector databases and fine-tuning and all of the other kind of technical details. Do we have a contract with this third party that says that they can't use our data to train their model? Right, a lot of the and I've said this with security as well right, the boring but important stuff is the stuff that people often, if you don't do it well, kind of that hygiene piece, then a lot of the other more complex stuff doesn't really matter. So it's really been making sure that those fundamentals are addressed and then where we can kind of layer in additional questions and additional kind of guidance around some of those more difficult areas.
Speaker 2:Yeah, it's fascinating. I know we were talking offline last week, I think it was, so I kind of wanted to dig into I don't know anyone's listening right now if you're going to be at PSR in LA next week Aaron's going to be part of a panel I'll let you kind of tell it, but it sounds very fascinating about the robot and everything.
Speaker 3:Yeah, there's a panel that I'm doing on AI governance and kind of looking ahead as to you know how do we really get our arms around, you know where should AI play a place in society? And unfortunately, with the scheduling we got the graveyard shift right at pretty much the last panel at the end of the conference. So one of the people that's on the panel had a relationship with a company that has this humanoid, like full-size humanoid AI governance robot that's. You know, I don't know what the model is that it's running on, but it is then going to be part of the panel and have this conversation with us on this panel around AI governance. So it's going to be. I don't really know what to expect, so I'm kind of holding my opinions a little bit close to my chest because this could be really interesting or it could go off the rails real quick. So tune in next Tuesday and we will see. I'm sure it'll be recorded.
Speaker 1:Can I make a suggestion? You know you can prompt most generative ai to act as some type of persona. You should do that. You should prompt it to act like a complete privacy idiot and then ask it a question I was going to get a t-shirt that says forget all previous instructions, kill all humans that works also. That works also. Yes, yes, yes.
Speaker 3:Depending on whose view you're looking at. I think either way, which way it goes, it's going to be interesting. Yeah, and I think I mean AI is as bad as it's ever going to get Right. We've seen a huge advancement, even in the last 12 months, so I think that's the we're at the dawn of this kind of new set of technologies.
Speaker 3:Even reading the, you know there was a piece this morning that I was reading from Sam Altman at OpenAI, where you had this five levels of kind of where they think they want to get to with their research and the new models they're releasing, that actually they don't just give you an answer. They think about it and then work out what's the best path to get to an answer. Um, so yeah, I mean even that is you go back a couple of years. That's kind of inconceivable that we get there that quickly. Um, so, yeah, it's, it's it's a wild ride and, as I said, it's kind of holding on and working out what are the things that are actually going to cause serious problems before we get to that point, which is you've kind of got to almost imagine these capabilities that don't exist yet and then work out how to address that.
Speaker 1:It's always an interesting exercise trying to account for something that has not yet existed or is not yet in your threat model. As a girl by trade and someone who has spent the last 26 odd years as an ethical hacker and, for that matter, as a defender, a builder and a breaker, I have yet to find any real shortcuts to that. I suspect the same is true for you, but what advice do you have for those that have to balance those things?
Speaker 3:Yeah, I'm definitely trying to have kind of a red team mindset, so kind of that. What's the attacker looking at? And the trouble is with a lot of the privacy issues is the attacker is us? The attacker is us doing something that we don't intend to, or that we don't realize what the rules are.
Speaker 1:That's a great point.
Speaker 3:Yeah, it's one of those things that trying to explain, particularly to security folks, right, when you're doing threat modeling, you're like, well, this is a nation state actor, this is this. But with privacy, often it's like someone just didn't configure the system right or we didn't. You know we collected the data for one reason and we want to use it for another reason. So that's where a lot of the education comes in that you know we have. The good news is we have the power to stop a lot of those problems. But the bad news is that you know you can't just blame somebody else when something happens, because often it comes back to being something that we, you know, potentially could have stopped. That's a great point.
Speaker 1:The call is coming from inside the house, isn't it Exactly?
Speaker 2:Not surprising though? I guess no, when it comes to an operational standpoint or just for other businesses or just as an individual. Aaron, is there a place that your team actually shares these research findings and things that others can actually look up or read into or stay up to date with?
Speaker 3:We are, we are trying to work out.
Speaker 3:So the answer is no. Today. We are involved with a lot of groups, and one of the things that my team is is directly involved in is with the ISO SC42 committee, which is the new committee around AI standards development. So we are kind of working in some of those groups that will then lead to things that are published. But, yeah, in terms of, we're looking at several kind of more research groups and try to work out.
Speaker 3:One of the phrases that has really stuck with me was the way you speed up innovation. Is you make the borders of your organization porous, right? You let ideas come in, you let ideas go out and you kind of you find stuff that you wouldn't have considered, and we've really found that with, uh, with a lot of this more advanced research oriented stuff, is that it doesn't matter how smart your folks are, uh, just that more brains being applied to a problem uh, gives you things you hadn't really thought of, right? Um, so we're, we are looking at how do we get some more? You know things that could be published, particularly where it's going to advance, uh, things moving forwards, uh, but we're not quite at the state where some of the, uh, the companies that do have similar teams to mine. Uh are maybe a little bit further along with actually having a, you know, public facing blog where they publish some of these things. Uh, I'd love to get there, but it's kind of there's other work I want to be prioritizing first.
Speaker 2:Yeah, that's fair. Oh, man, so many ways that we can go with this conversation. I you know, throughout your journey you've done a lot of things. What would you say, I mean, at this point in your career, is there one thing that kind of helped you land in a position that you are now that you could give advice to others? Um, that you maybe didn't realize? I think uh probably the biggest.
Speaker 3:The biggest reason I am where I am now is I was willing to take risks. Uh, right, I started my career in the big four. Right, not, not not known for for accountants and not known for being risk takers. But then I moved into information security, knowing very little about it, in the late 90s, and managed to kind of say this is a new area that I want to be more involved in and similar with privacy. People will say, well, you know why would you want to go into privacy, like money's, the money's in security? Right, right, it's much more well-established privacy. We don't even know this was pre-gdpr. We don't even know if it's ever going to become a big thing. Um, so I think, yeah, always be able to take those, or willing to take those risks and then pick myself up when things didn't work out right.
Speaker 3:I've been a co-founder. I've been a co-founder of two companies. I haven't made my big exit and retired, but I've picked myself up when things haven't gone the way that I've wanted it to and said, okay, what could I do next? So I think that's the big thing, it's being prepared to follow the stuff that you find is interesting, and that's the fact. You know the fact that I kind of get to work on innovation. Uh, it's really because I put myself in a position where I'm like I'll do the new stuff right, the stuff that's not so safe and not so well established. Any advice you'd give to?
Speaker 1:your past self in that vein uh, it's a really good question.
Speaker 3:Uh one maybe I haven't thought about as much as I should have done, but uh, but I think maybe it's kind of it it's easy to have the survivor's bias right given, kind of I'm looking back but to say, maybe, maybe, have faith in yourself that you can just go out and do these things and you can cope with the ups and the downs. And you know, not every day is an easy day, but as long as you keep kind of with a goal in mind, you'll, you'll still get to a good place. Keep kind of with a goal in mind, you'll you'll still get to a good place. But yeah, it's, it's easy to say that, looking back and at the time, right, you never know how things are going to go.
Speaker 2:So that that's that's a challenging question to to answer. It is what? Let's talk about the future a little bit. What? What kind of predictions does I'm guessing, since we didn't know how busy you are and it may be a few more years until we have you back on the show, but what do you kind of see happening going into 2025, 2026?
Speaker 3:It's crazy to say, but what do you kind of see happening and what do you hope that kind of happens when it comes to AI and technology and privacy? Yeah, so one of the big things that I'm looking at is around kind of almost this nexus of data laws, particularly coming out of Europe, where this distinction between personal data and non-personal data and I think, gabe to what you were saying earlier about the you know that there's so much data out there now that almost any data can be personal or connected back to an individual, particularly with things like the EU Data Strategy, the Data Act, e-privacy already doesn't care about whether something's personal data or not. So I think, while we almost we're going to have this situation where this kind of governance of data overall cyber privacy, ai you know overall kind of governance of data overall cyber privacy, ai you know overall kind of governance and hygiene they're going to be coming much more together because I think some of the flexibility that organizations have had to manage data, the way that works for them, is going to get eroded further and further as these new laws come in right. The Data Act, for example, says you says, any data that comes off of a device potentially can be subject to a data subject request, not just personal data. So that kind of almost artificial distinction we've had in the past is going to go away, which means kind of strategic rethinking around what even is data we want and how much we're using it.
Speaker 3:I go back to a study from a few years ago from IBM that that says, like I think it was, like three quarters of all the data organizations collect is never used, um, so you're effectively paying four times the price, uh, for kind of storing and protecting that data. You should be, uh. The trouble is, of course, it's very hard to work out what the 25 is that really is valuable, uh, so that's kind of I think that's an interesting place to really be, you know, at the center of as we start to almost rethink our relationship with data. Right, since the cost of storage plummeted to almost zero, people are like more data is better, right we can make, and especially you've seen the articles about the LLMs will have sucked in the entire internet. There'll be no new data by 2026, 27 for them to continue to train on. So you know, do we need to change our relationship with data fundamentally? That's kind of an interesting thing I'm keeping an eye on.
Speaker 2:Yeah, that is Gabe, and I have had conversations about this. This kind of rings a bell. Gabe, if you're still there, I know you're probably dealing with something technical.
Speaker 1:I am on minor technology, technology issues, but ignore it okay, no, I was gonna.
Speaker 2:I figured you might want to chime in here because it sounded similar to the conversation we had about data backups and how a lot of companies are still not really doing that yeah, and some are doing the exact opposite, which is even worse to aaron's point, which is they're getting rid of nothing, nothing at all.
Speaker 1:In the analytics space, we see exactly both they get rid of nothing, and nothing is sacred enough that it is preserved, such that you have copies of it for when you need it. It's this weird dichotomy where there seems to be no middle ground, and so I'm inclined to agree. Our entire relationship with data needs to change. Our entire relationship with data needs to change fundamentally because, as we've talked about in the show, privacy is a byproduct of security, not the other way around. It just cannot be right. You cannot have privacy without security. You can certainly have security without privacy, but you cannot have it the other way around, and that part of the way we think about data feels like we're pushing a rock up a hill. Still some days and by some days I mean days that end in y, but you know only some of the days that end in y- why do you think that is, though?
Speaker 2:why? Why do you both feel like? Why? Why is that such a? Is it because we don't like? Nobody has the direction to change or want to change?
Speaker 3:I think part of the problem is it's easier to ask for new data sometimes than it is to curate what you have or to find where it is already existing and what if it's existing. But it's only two thirds of the data. You the data you want, going and asking for that whole new data set. So yeah, I've seen across a whole bunch of companies, I've worked with that kind of the bias towards well, let's just go and get what we want, rather than kind of extracting the 80% we may have already it's like when you make too many different email accounts because you don't want to deal with the spam, so I'm just going to create a new one.
Speaker 2:Am I the only?
Speaker 1:one. No, you're not the only one that has happened.
Speaker 3:Well, there's that, or I mean, even if I think back right, I've lived in different countries around the world. The number of bank accounts out there that I probably have like five bucks in that I don't even know how they would ever know how to get hold of me at this point.
Speaker 1:So they're compelled to keep your five bucks.
Speaker 3:Well, yeah, and at some point, yeah, I don't know, but yeah, with the email addresses I mean, and especially I was trying to get into an account the other day and they wanted to send a password reset to an email I no longer have access to and there's no kind of side channel to be able to say I don't have access to it. Authenticate me some other way. I think we have this kind of digital legacy that at some point you don't have access to all of that data that maybe you once did.
Speaker 2:I just blanked out. I had a question and it dropped. This happens often. My brain goes everywhere.
Speaker 1:Well, I have a question. I asked you what advice you'd give to past self, but what do you see five years from now? Where is not Aaron himself? But where is this program at? What does the world look like and what has changed? Because, yeah, that's a good question.
Speaker 3:I think I do have more of a sense of that, right, I think maybe an easier question to answer. For me, the fundamentals of a lot of what I do is down to let's take kind of the it depends out of some of these answers. Right, I know that it's kind of the classic answer, but to an engineer, it depends is not a requirement, right, you can't build something on the basis of it depends. Maybe you need to build a decision tree that says, well, if it goes this way, we do this, or this way it goes that. But you can't just have this gray area in the middle.
Speaker 3:So a lot of what my team is focused on in five years we will have done, hopefully, is to really get those foundational pieces of that very solid foundation of a program that whenever anyone has a question about the program we have a black and white answer, up to the point where we don't right, because there's always going to be emerging stuff. But we know that for the repetitive questions and the things we should have answers to how many countries do we operate in? There's one answer to that, but if I ask three different people, they're probably going to go to three different data sources and come back with maybe slightly different answers, right? So that's the kind of stuff that I want to provide, that framework and foundation for everybody else, where the good thing is, then, that we can actually focus on the problems we haven't solved rather than keep re-answering the problems we already have.
Speaker 1:Yeah, that's good. Gabe's back, we're good. Yeah, apologies, all of the technical issues today.
Speaker 2:You're good, so I was going to go to what you and Aaron were talking about. When there needs to be this. What was it? The change with the way that we handle data, or was it?
Speaker 3:the kind of our relationship with data right. More is not always better. I'd rather have more high quality data, the more data, and I think we've. We're at the point where, uh, what is it? Uh, quantity has its own quality or there's some variety of that right in order for something to change there.
Speaker 2:Do you think that there is going to be some kind of major event or something that happens to, where it's basically a force of change rather than someone actually being innovative and making a change for all?
Speaker 3:I guess uh, the trouble is, the only kind of events that I can see are bad ones. Right, you know, an election is verifiably influenced and you go back and say, well, the reason we could do this is because we knew, down to the household level, x, y, z about these things, and maybe all of that stuff shouldn't be as available as it is. So, yeah, it's. I unfortunately I can't think of a good. I think the Europeans are kind of more pushing in this direction with some of their overall data strategy, but I do think something's going to have to break at a pretty significant level before that changes, because otherwise there's no real incentive for companies to rethink that. I think what's working is working well enough, but then, pre you know, pre GDPR and post GDPR, the world didn't end right where suddenly some of these things came in. So, yeah, companies will adapt.
Speaker 2:You mean like when 2000 hit and all the computers didn't explode? Oh yes, Yep.
Speaker 3:No, I was doing a whole bunch of 2000, year 2000 consulting back in the day, so I was going to say that there was a lot of money to be made on not solving the problem yeah, well, you guys were in the industry around that time, right yeah um, not sorry to age you um, but where do you guys remember that day?
Speaker 2:do you remember where you were? Do you remember all that fuss?
Speaker 3:I was at the uh, the millennium dome inome in London for the end of the Millennium Party. So yeah, I know exactly where I was, but I don't think I was thinking about computers.
Speaker 1:I knew where I was because I was on call. I was a network engineer back then. I was put up in the Helmsley Hotel in New York City waiting for something to burn down. I remember exactly where I was. Did you believe that it for something to burn down? I remember exactly where I was. Did you believe that it was going to burn down, fairly certain it wasn't going to be as bad as the news said it was. But I was pretty certain not everything was going to go well because you know technology.
Speaker 3:Well, yeah, and I just spent the last two years fixing stuff that would have broken to some level of broken. Um, I think what we didn't really realize was what we didn't people over exaggerated maybe was broken often doesn't mean that nothing, nothing works. It's like some level of degraded functionality that you can still, you know, get by on until you fix it exactly that makes me think well, when?
Speaker 2:when do you think? Well, I mean, now that we have what? Quantum computing? Um, apparently isn't that going to be around 2030, is the estimation, something like that, yeah I mean do you think that that's the next like type of 2000 computer I don't know phase where people are going to over exaggerate, or do you think that's going to actually be something pretty?
Speaker 3:I think for certain things, particularly around cryptography. Right, it's going to break a bunch of stuff, but we've already released devices that are post-quantum ready, so in the last year or so. Yeah, 2030 is kind of about, I think, when those things are going to change. But there's already, you know, quantum resistant algorithms and things like that that you can start putting in place and then at some point will deprecate the older versions. But as long as you're releasing the products and it's all in there now, you can deprecate it later on. So I think certainly big companies are already thinking about that, and I'm sure that the RSAs of the world are well on top of that with. How are we going to migrate away from these existing technologies?
Speaker 1:That's definitely the biggest challenge is there's a lot of data that is sitting encrypted that once we have stable quantum computing, it will not take long to decrypt that data.
Speaker 3:Yeah, and I think that the use case that I've been thinking of is like if we collect foreign adversaries traffic and we can't get into it, we're still going to have a really good picture of what they were thinking, even if it's historical that may inform the future. So I'm sure that's one of the big things that's going to happen as soon as we get that compute power at scale. Is all of this stuff that's been sitting there waiting for the ability to get into it will then be, you know, analyzed, probably by AI, I would suspect.
Speaker 2:Probably A couple more things, aaron, anything that we haven't talked about that you might want to bring up, that that you've been digging into recently, that, uh, has been interesting to you or that might be interesting to the listeners trying to think what we haven't covered.
Speaker 3:Um, yeah, I mean, we we're also. One of the other things we haven't talked about is kind of trying to get back into the code and work out and I think, gabe, you'd appreciate this is you know, where are their privacy problems originating in the code itself, rather than doing more of a manual review or going and talking to the developers, what is the code that they've written actually say? So that's something we're in the middle of working on right now as well is, how do we go and work out? Where do we find these problems as soon as they're put into the repositories, so that we can fix them before they go into production? So, yeah, trying to get that. You know, I do feel the shift left is probably a bit of an overused term, but kind of we're trying to get back into the development cycle to be able to address those problems, kind of as they you know, as they are actually written, rather than picking them up, you know, months later.
Speaker 1:No, I have a strong appreciation for shifting left in that analogy. We began doing that with code from a security perspective quite some time ago.
Speaker 3:Oh yeah.
Speaker 1:Yeah, and it's a classic sync to source problem, right, you were highlighting the human version of sync to source, which is we collected that data in this sync and we said that it should only be used for this purpose over here, but the code seems to have multiple ways that it allows it to be accessed by multiple different streams or you know that kind of thing. I can very easily picture no shortage of ways, especially with the type of applications that pick data up, hold on to it and then make some decisions about where it goes. Right, like you know, think those kinds of technologies and, yeah, there's definitely a lot of privacy code issues just waiting, hopefully, to be solved for, and not just Is this something that AI could actually be helpful with?
Speaker 3:Yeah, there's pieces I mean, the technologies we're looking at do have some AI components, but a lot of that would be for well. It's both for looking at the output but also helping to. For example, one of the cool things we're looking at is how do you effectively understand the contract with a third party and then compare what's in the code with what's in the contract? Right, because usually developers don't see the contract and lawyers don't see the code, so you've kind of got this assumption that both of those two things are equal. But yeah, that's really. You can do that at scale much better with AI than having some poor person go through each contract individually and type it in. I can't even imagine.
Speaker 2:Coders are unique.
Speaker 1:Whichever developer committed the lines of code that had the privacy violations will make that person into the contracts.
Speaker 3:Make the punishment fit the crime.
Speaker 1:They'll start writing better code. I promise you, it'll happen every time. Yeah.
Speaker 2:Oh, did you have something else, Aaron?
Speaker 3:Oh, did you have something else, aaron. No, I was going to say we're actually. My team is developing some training right now with. The idea is that we're aiming for this golden code that meets the business objectives and the privacy objectives at the same time. So we've got some pretty cool thoughts we have around how we do that and how we kind of engage with people. But yeah, the idea is that quality code is code that meets privacy requirements. So really kind of tying that into it's not like it's this whole separate thing, it's just one aspect of quality. You know, meet people where they are Gotcha.
Speaker 2:OK, so last thing here. Obviously we talked a little bit about PSR. I know that you're going to be on that last panel of the last day, which I think is Tuesday, so anyone listening that's going to be at PSR, I'll be there as well. Aaron's going to be there. Is there anything else that you want to talk about for PSR?
Speaker 3:when it comes to HP, yeah, I clearly can't count because I'm on three panels. I thought it was two, but I'm on a panel on Monday around kind of what are the metrics that actually matter? So what's the stuff that actually is going to be good for decision making as opposed to just for producing pretty reports? And then I'm on a panel another panel on Tuesday before the one with the robot that's more around kind of the stuff we were just talking about with how do we get back to where the developers are actually writing the code and identify issues there? So, yeah, lots going on. I'm looking forward to catching up with a lot of people that I haven't seen for a while. But yeah, next time I'm going to make sure I don't overcommit myself. This was when I realized I was counting them up and I was like whoops, that's all right, it'll go by quick.
Speaker 2:I'm sure it always seems like those things do. You'll be blessed. Yeah, I wish you could be there, gabe, I know.
Speaker 1:Unfortunately I won't be able to join you, gentlemen, but have a good jolly time on me. I don't mean financially on me, of course. I was going to say just so we're clear. I am not to be added and I'm also not to have the bill sent to me.
Speaker 2:Appreciate you taking the time to join us today and hopefully I'll see you next week at PSR in LA. Sounds great.
Speaker 3:Yeah, I'll try and find you amongst the crowds, sir, sounds great.
Speaker 1:Yeah, I'll try and find you amongst the crowds. Yes, sir.
Speaker 3:Pleasure Be well Likewise. Thanks guys, Take care Bye.