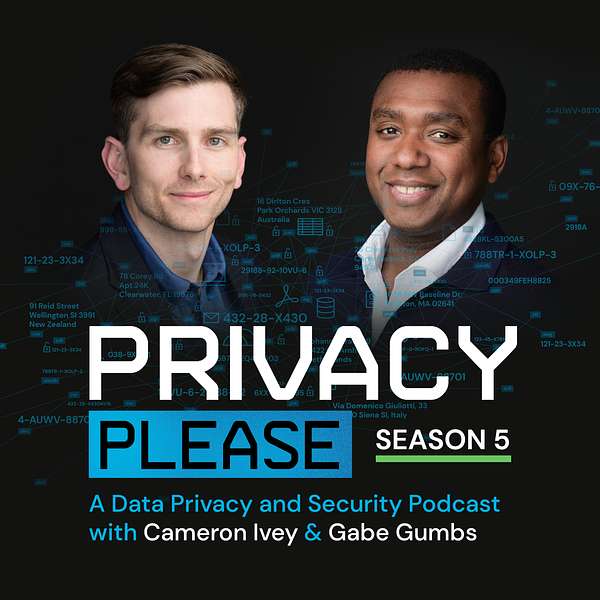
Privacy Please
Tune into "Privacy Please," where hosts Cam and Gabe engage with privacy and security professionals around the planet. They bring expert insights to the table and break down complicated tech stuff everyone can understand.
Privacy Please
S5, E205 - Exploring the Privacy & Cybersecurity Risks of Large Language Models
Prepare to have your mind expanded as we navigate the complex labyrinth of large language models and the cybersecurity threats they harbor. We dissect a groundbreaking paper that exposes how AI titans are susceptible to a slew of sophisticated cyber assaults, from prompt hacking to adversarial attacks and the less discussed but equally alarming issue of gradient exposure.
As the conversation unfolds, we unravel the unnerving potential for these intelligent systems to inadvertently spill the beans on confidential training data, a privacy nightmare that transcends academic speculation and poses tangible security threats.
Resources: https://arxiv.org/pdf/2402.00888.pdf
It's all good. Ladies and gentlemen, welcome back to another episode of Privacy, please. I'm your host, along with Gabe Gumbs. We are here hanging out. It's Tuesday.
Speaker 2:I almost said Monday.
Speaker 1:But having a good week so far. Gabe, how are you doing today?
Speaker 2:I'm solid man. I'm decent, Doing all right. I wonder how everyone out there in the privacy and security world is doing. How about you? How do you feel? Doing all right. I wonder how everyone out there in the privacy and security world is doing? How about you?
Speaker 1:How do you feel? I feel real good. Oh, all right, hey, waking up, breathing, trying to stay fit, better than waking up, not breathing, that's for sure. Yeah, you don't want to not breathe no staying fit's good.
Speaker 2:Some crazy things have been happening around the world Just as I'm thinking. Did you see that bridge in baltimore collapse from I heard? I heard about it this morning like I was. I was on my way to to grab some tea and I turned on the radio and I was like I'm sorry, did you say the entire bridge went into the? Uh?
Speaker 1:river. Is that, yeah, terrifying. Um, I'm pretty sure some people definitely died. So because I think that there were cars on the bridge, yeah, uh, scary. I feel really bad for those people and their families and stuff. That's got to be so because.
Speaker 2:I think that there were cars on the bridge. Yeah Scary. I feel really bad for those people and their families and stuff that's got to be.
Speaker 1:That is an awful thing to wake up to. I don't want to go on a bridge now.
Speaker 2:I mean I know obviously the ship ran into it and then took it down and collapsed it, but like could you I have a relatively irrational fear of bridges also to be like, to be transparent, like always, like every time I drive over and I'm always like okay yeah what was that myth busters episode like how do you get out of this car if it goes into the water again?
Speaker 1:you. You roll your window down or bust it open and you get out before it goes underwater before it submerges.
Speaker 2:That's the yes, that's the key. You got anybody in?
Speaker 1:the back. You better fend for yourself and get.
Speaker 2:Well, no, I'm just kidding anybody in the back. I hope you're also listening to this.
Speaker 1:Yeah, yeah, get out immediately before it actually submerges, because obviously it becomes way harder to get out. Yeah, yeah, yeah, yeah. So don't worry about stuff that's in the car, just worry about your life. Those are materialistic things. Get out of there.
Speaker 2:It does put things into perspective. We cover a lot of dangerous topics on this show, but nothing quite as dangerous as those real-life events.
Speaker 1:No, but I mean rolling into this. You had shared with me something that uh found pretty fascinating right now. Um yeah, this, this paper that a bunch of different people wrote security and privacy challenges of large language models. What are you getting from this? Let's, let's get a high level.
Speaker 2:Yeah, so the listeners so, first of all yep, that's the name of the paper Security and Privacy Challenges of Large Language Models a Survey. It was just recently published by a few folks, so shout outs to Bharan Chandra, Hari Amini, Yanzhou and the Florida International University. Fiu is probably the most comprehensive I've seen so far of security research that specifically looks at large language models and the vulnerabilities that exist, from both the security and the privacy perspective. I think, it's extremely timely. I've dabbled, I've more than dabbled.
Speaker 2:I've spent a significant portion of my career as a hands-on ethical hacker and I still dabble in that area. But a lot of folks in my circle are still very active. You know penetration testers, red teamers, blue team, purple team, you name it. Uh, a lot of folks very close to me still in that world, and a topic that's been coming up frequently amongst them and us is how do you test ai platforms, including large language models, and and a lot of the folks I've spoken with have largely responded with there's no. No one really has an answer right now.
Speaker 2:A lot of folks are approaching testing from a traditional place of how you examine the boundaries of systems, but this paper in particular gets really deep. So it looks at the security and privacy threats and it discusses specific attack types. The security and privacy threats and it discusses specific attack types, everything from prompt hacking to adversarial attacks. One of the ones that I'm personally looking forward to digging into a little bit more are gradient exposures. So you know, gradients are ways that training data can be shared. I mean, I previously thought to not expose private training data from publicly shared gradients. So there's like some huge security privacy concerns there, too, where you may have trained the model on what may have been de-identified data and, through a gradient further try to not expose that private training data.
Speaker 2:And there's a wealth of real interesting research that further looks at the real challenges that LLMs are faced, versus the theoretical challenges that we oftentimes discuss. So it's a hell of an interesting paper and I know we're going to cover it a little bit more. One of the things that we're going to do is we'll get into a blog cast on it, but we'll look to get some folks on the show that can really dive into these topics in a bit more depth. I would fast start exceeding some of my own capabilities of understanding at the moment, um, if I tried to get too deep into those waters. But uh, I plan on cozying up further with this document. It's interesting it is.
Speaker 1:Um, I can't wait to dig into it a little bit more and, like gabe said, um, after this, very shortly, if you stay tuned, it will be followed by a, a nice blog cast that'll kind of go a little bit more in depth, and then from there we'll uh, we'll try to bring on some of these people that actually were part. It will be followed by a nice blog cast that will kind of go a little bit more in depth, and then from there we'll try to bring on some of these people that actually were part of this so we can dig even further Right on.
Speaker 2:I think it will be amazing. Yeah, other than that, that's pretty much it for this. This is a chunky one. I think we both agree that this week we really wanted to share this with the uh, with our, our audience. Um, and let it kind of simmer a bit more and and we'll we'll dive into this topic quite heavily on our next episode nice.
Speaker 1:well, stay tuned for the broadcast and gabe. Thank you, sir. We'll see you guys next week right on peace, peace out. Thanks for hanging around. Let's go ahead and dive into this.
Speaker 1:In the era of ever-expanding AI capabilities, large language models, also known as, say it with me, llms stand at the forefront of innovation. Stand at the forefront of innovation From generating human-like text to powering virtual assistants. These models have revolutionized various industries. However, amidst their remarkable potential lies a complex web of security and privacy challenges that demand our attention. Recently, a paper titled Security and Privacy Challenges of Large Language Models a survey shed light on these critical issues. Authored by Badhan Chandra Das, m Hadi Amini and Yan Zahu Wu from Florida International University, the paper provides a comprehensive analysis of the vulnerabilities inherent in LLMs and proposes robust defense strategies. It's fascinating. Let's go ahead and dig in a little deeper, understanding the threat landscape. And before we dive into that, I just wanted to thank all of these individuals and the Florida International University for putting this all together. It's amazing. I'll share the graph. It's very intensive and very thorough. It's a lot of pages. So LLMs, despite their prowess, are not immune to security breaches. The paper categorizes the threats into three main types prompt hacking, adversarial attacks and privacy attacks. Prompt hacking involves unauthorized access to the model's prompt mechanism, while adversarial attacks aim to manipulate the model's behavior through poisoned data. Privacy attacks, on the other hand, target the leakage of personal identifiable information, also known as PII. Very good, very good. Now let's talk about implications across all industries. The implications of these security threats extend across diverse sectors, including transportation, education and healthcare. For instance, compromised LLMs could lead to misinformation in transportation systems or jeopardize patient privacy in healthcare applications. Understanding these risks is crucial for safeguarding sensitive data and ensuring the integrity of AI-driven systems.
Speaker 1:Defense strategies a multi-faceted approach To combat these threats effectively. The paper proposes a multi-faceted approach encompassing various defense mechanisms. Let's go over some of those right now. So the first one is prompt injection prevention. Techniques like paraphrasing and retokenization are employed to detect and prevent prompt injections. Integrity checks on data prompts help identify potential compromises, bolstering the model's resilience against attacks.
Speaker 1:Number two is jailbreaking attack mitigation, processing and filtering techniques are utilized to block undesired content, reducing the likelihood of successful jailbreaking attempts. Strategies such as self-reminders and key flagging aid in identifying and neutralizing potential threats. Backdoor and data poisoning attack detection is number three. Methods like fine-tuning and model pruning are employed to counter backdoor attacks. Clustering algorithms help distinguish between poison and clean data, enhancing the model's robustness against manipulation. Number four is privacy preservation strategies. Defense against privacy attacks involves data violation and validation, anomaly detection and limiting training epochs to guard against data poisoning. Identifying and filtering out poisoned examples are essential steps in protecting sensitive information.
Speaker 1:Number five gradient leakage and membership interference. Attack mitigation Techniques such as adding noise, applying differential privacy and homomorphic encryption help thwart gradient-based attacks while preserving utility. Dropout. Model stacking and adversarial regularization are employed to mitigate membership interference attacks, reducing the risk of overfitting and enhancing generalization. And number six PII leakage prevention. Pii leakage prevention Our strategies focus on minimizing memorized text and removing PII through deduplication and scrubbing techniques. The use of differential private stochastic gradient descent, which is also known as DPSGD, during pre-processing adds an additional layer of protection against PII leakage.
Speaker 1:Let's kind of wrap all this up In conclusion toward a more secure AI future. In the age of AI-driven innovation, addressing the security and privacy challenges of large language models is imperative. I think we have learned by understanding the vulnerabilities and implementing robust defense strategies. We can harness the full potential of LLMs while safeguarding against malicious actors. The insights provided in this paper serve as a roadmap for researchers and practitioners alike, guiding the development of secure and privacy-preserving AI systems across various domains. Ladies and gentlemen, as we navigate this ever-evolving landscape of AI, collaboration and vigilance will be key in ensuring a secure and trustworthy future powered by large language models.
Speaker 1:Ladies and gentlemen, that is the end of today's episodes. Thank you so much for tuning in. Hope you liked it. If you have questions, I'm going to send or I'll have the link to this entire graph and everything that you're going to want to if you want to dig even deeper into it. But hopefully that gave you a good high level overview of this and love what we're doing here, love the integration of privacy and security in this and can't wait to see what happens. So thanks again for tuning in and we'll see you guys next week. Cameron Ivey, over and out.